NeRFs represent 3D scenes in a neural network. A new paper by an international team including ETH Zurich opens up AI technology to dynamic scenes.
In summer 2020, Google researchers presented Neural Radiance Fields (NERF) is planning to launch a AI-process that can extract 3D depth data from 2D images such as photos. NERF can thus create a textured 3D model from multiple photos shot from different angles. Neural Radiance Fields (NeRFs) can thus render 3D scenes from previously unseen angles. This enables, for example, a 360-degree camera tour around an object, a tour via drone shot or a flight through the interior of a restaurant. The technology can thus be used to generate photo-realistic 3D objects.
In almost all cases, however, these are static scenes or objects, since movements introduce a temporal dimension into the training process that has been difficult to resolve so far.
NeRFs for dynamic scenes
In a new research paper, a team from the University at Buffalo, ETH Zurich, InnoPeak Technology and the University of Tübingen now shows how NeRFs can represent dynamic scenes and thus learn a 4D representation.
RGB images from different cameras or a single moving camera serve as input. The images show people moving or someone pouring coffee into a glass.
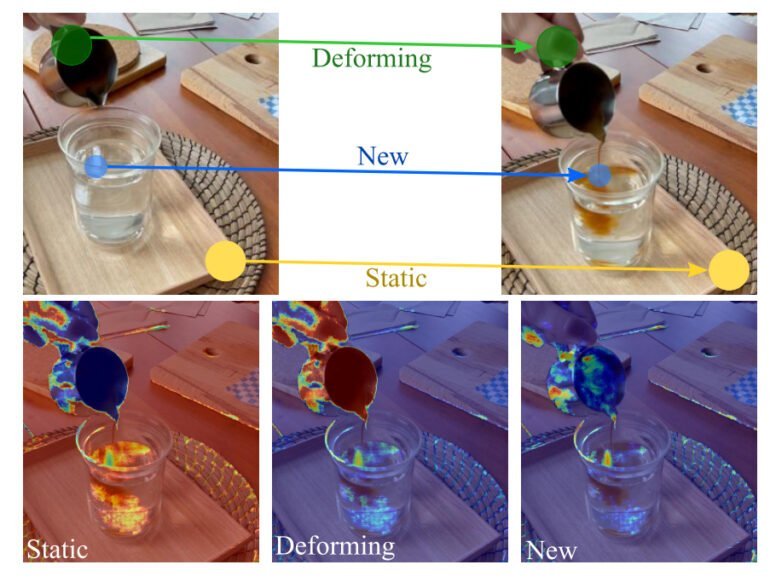
In the case of the coffee, for example, the wooden board on which the glass stands remains static. The contents of the glass appear anew and the visible hand deforms. A decomposition field takes over the division of the scene into the three categories. Each area is represented by its own neural field. In their approach, the researchers also decouple temporal and spatial dimensions to improve the representation.
Virtual reality as a vision
The decompositional representation of the dynamic scene significantly reduces visual artefacts compared to other approaches. The team also demonstrates with NeRFPlayer a way to stream the learned representations in real time with limited bit rates.
Through Nvidia's framework InstantNGP, with which a neural network can learn representations of gigapixel images, 3D objects and NeRFs within seconds, the method presented is also fast.
In the work, the team describes the visual exploration of a real 4D spatio-temporal environment for virtual reality as a vision and sees their own work as a contribution to this goal.
"Visual exploration of a real 4D space freely in VR is a long-standing task. The task is particularly appealing when only a few or even a single RGB camera is used to capture the dynamic scene," the paper states.
The NeRF demonstrations have their origins in a Google research paper from 2020 on spatially filmed light field videos.